Up Glossary Topics What stat? Nonparametric Links SPSS
Simulation & Gaming:
An Interdisciplinary Journal
+++
| |
PROPHET StatGuide: Glossary
Taken from http://www.basic.northwestern.edu/statguidefiles/sg_glos.html
- alternative hypothesis:
- The null hypothesis for a statistical test
is the assumption that the test uses for calculating the probability of
observing a result at least as extreme as the one that occurs in the data at
hand. An alternative hypothesis is one that specifies that the null
hypothesis is not true.
For the
one-sample t test, the null hypothesis is that the
population mean equals a specific value. For a two-sided test, the
alternative hypothesis is that the mean does not equal that value. It is also
possible to have a one-sided test with the alternative hypothesis that
the mean is greater than the specified value, if it is theoretically
impossible for the mean to be less than the specified value. One could
alternatively perform one-sided test with the alternative hypothesis that the
mean is less than the specified value, if it were theoretically impossible for
the mean to be greater than the specified value.
One-sided tests usually have more power than two-sided
tests, but they require more stringent assumptions. They should only be used
when those assumptions (such as the mean always being at least as large as
they specified value for the one-sample t test) apply.
- between effects:
- In a repeated measures ANOVA, there will
be at least one factor that is measured at each level for every subject. This
is a within (repeated measures) factor. For
example, in an experiment in which each subject performs the same task twice,
trial (or trial number) is a within factor. There may also be one or more
factors that are measured at only one level for each subject, such as gender.
This type of factor is a between or grouping factor.
- bias:
- An estimator for a parameter is unbiased if its expected value is
the true value of the parameter. Otherwise, the estimator is biased.
- binary variable:
- A binary random variable is a discrete
random variable that has only two possible values, such as whether a subject
dies (event) or lives (non-event). Such events are often described as success
vs failure.
- boxplot:
A boxplot is a graph summarizing the distribution
of a set of data values. The upper and lower ends of of the center box
indicate the 75th and 25th percentiles of the data, the center box indicates
the median, and the center + indicates the mean. Suspected
outliers appear in a boxplot as individual points o
or x outside the box. The o outlier values are known as
outside values, and the x outlier values as far outside
values.
If the difference (distance) between the 75th and 25th percentiles of the
data is H, then the outside values are those values that are more than
1.5H but no more than 3H above the upper quartile, and those values that are
more than 1.5H but no more than 3H below the lower quartile. The far outside
values are values that are at least 3H above the upper quartile or 3H below
the lower quartile.
Examples of these plots illustrate various situations.
- cell:
- In a
multi-factor ANOVA or in a contingency table,
a cell is an individual combination of possible
levels (values) of the
factors. For example, if there are two factors, gender with values
male and female and risk with values low,
medium, and high, then there are 6 cells: males with low risk,
males with medium risk, males with high risk, females with low risk, females
with medium risk, and females with high risk.
- censoring:
- In an experiment in which subjects are followed over time until an event
of interest (such as death or other type of failure) occurs, it is not always
possible to follow every subject until the event is observed. Subjects may
drop out of the study and be lost to follow-up, or be deliberately withdrawn,
or the end of the data collection period may arrive before the event is
observed to happen. For such a subject, all that is known is that the time to
the event was at least as long as the time to when the subject was last
observed. The observed time to the event under such circumstances is
censored.
Survival analysis methods generally allow for censored data. Censoring may
occur from the right (observation stops before the event is observed), as in
censorship for survival analysis, or from the left (observation does not begin
until after the event has occurred).
- central tendency:
- The generalized concept of the "average" value of a
distribution. Typical
measures
of central tendency are the mean, the median, the mode, and the geometric
mean.
- centroid:
- The centroid of a set of multi-dimensional data points is the data point
that is the mean of the values in each dimension. For X-Y data, the centroid
is the point at (mean of the X values, mean of the Y values). A simple linear
regression line always passes through the centroid of the X-Y data.
- chi-square test for goodness
of fit:
- The chi-square test for goodness of fit
tests the hypothesis that the distribution of the
population from which nominal data are drawn agrees
with a posited distribution. The
chi-square goodness-of-fit test compares observed and
expected frequencies (counts). The
chi-square test statistic is basically the sum of the squares of the
differences between the observed and expected frequencies, with each squared
difference divided by the corresponding expected frequency.
- chi-square test for independence
(Pearson's):
- Pearson's
chi-square test for independence for a
contingency table tests the null hypothesis
that the row classification factor and the column
classification factor are
independent. Like the chi-square
goodness-of-fit test, the chi-square test for independence compares
observed and expected frequencies (counts).
The expected frequencies are calculated by assuming the null hypothesis is
true. The chi-square test statistic is basically the sum of the squares of the
differences between the observed and expected frequencies, with each squared
difference divided by the corresponding expected frequency. Note that the
chi-square statistic is always calculated using the counted frequencies.
It can not be calculated using the observed proportions, unless the
total number of subjects (and thus the frequencies) is also known.
- conservative:
- A hypothesis test is conservative if the actual significance level for the
test is smaller than the stated significance level of the test. An example is
the
Kolmogorov-Smirnov distribution test, which becomes conservative when the
parameters of the distribution are estimated from the data instead of being
specified in advance. A conservative test may incorrectly fail to reject the
null hypothesis, and thus is less
powerful than was expected.
- consistent:
- A hypothesis test is consistent for a specified
alternative hypothesis if the
power of the test for the alternative hypothesis
approaches 1 as the sample size becomes infinitely large.
- contaminated normal
distribution:
- A contaminated normal distribution is a type of mixture
distribution for which observed values can come from one of multiple
normal distributions. For example, in
taking measurements of blood pressure from a population, the distribution for
males may be a normal distribution, the
distribution for females may also be a normal distribution, but if the two
normal distributions do not have the same mean and variance, then the
composite distribution is not normal.
A common type of contaminated normal distribution is a composite of two
normal distributions with the same mean, but with different variances, such
that only a minority of the values come from the distribution with the larger
variance. Such a distribution is heavy-tailed
relative to the normal distribution. If the proportion of values from the
distribution with the larger variance is small enough, the contaminated normal
distribution may look like a normal distribution with outliers. In such a
situation, one should be alert to the possibility of a connection or common
trait among the outlying values that might suggest that all come from a second
distribution with a different variance.
- contingency table:
- If individual values are cross-classified by levels in two different
attributes (factors), such as gender and tumor vs no
tumor, then a contingency table is the tabulated counts for each combination
of levels of the two factors, with the levels of one factor labeling the rows
of the table, and the levels of the other factor labeling the columns of the
table. For the factors gender and presence of tumor, each with
two levels, we would get a 2x2 contingency table, with rows Male and
Female, and columns Tumor and No Tumor.
The counts for each cell in the table would be the number
of subjects with the corresponding row level of gender and column level of
tumor vs no tumor: females with tumors in row 1, column 1; females without
tumors in row 1, column 2; males with tumors in row 2, column 1; and males
without tumors in row 2, column 2, as shown in the picture. Contingency tables
are also known as cross-tabulations. The most common method of
analyzing such tables statistically is to perform a
(Pearson) chi-square test for independence or Fisher's
exact test.
- correlation:
- Correlation is the linear association between two
random variables X and Y. It is usually
measured by a correlation coefficient, such as Pearson's r, such that
the value of the coefficient ranges from -1 to 1. A positive value of r
means that the association is positive; i.e., that if X increases, the value
of Y tends to increase linearly, and if X decreases, the value of Y tends to
decrease linearly. A negative value of r means that the association is
negative; i.e., that if X increases, the value of Y tends to decrease linearly,
and if X decreases, the value of Y tends to increase linearly. The larger r
is in absolute value, the stronger the linear association between X and Y. If
r is 0, X and Y are said to be uncorrelated, with no linear association
between X and Y. Independent variables are always
uncorrelated, but uncorrelated variables need not be independent.
- covariate:
- A covariate is a variable that may affect the relationship between two
variables of interest, but is not of intrinsic interest itself. As in
blocking or
stratification, a covariate is often used to control for variation that is
not attributable to the variables under study. A covariate may be a discrete
factor, like a block effect, or it may be a continuous
variable, like the X variable in an
analysis of covariance.
Note that some people use the term covariate to include all
the variables that may effect the response variable, including both the
primary (predictor) variables, and the secondary variables we call covariates.
- curvilinear functions:
- A curvilinear function is one whose value, when plotted, will follow a
continuous but not necessarily straight line, such as a polynomial, logistic,
exponential, or sinusoidal curve.
- death density function:
- The death density function is a time to failure
function that gives the instantaneous probability of the event (failure). That
is, in a survival experiment where the event is death, the value of the
density function at time T is the probability that a subject will die
precisely at time T. This differs from the hazard
function, which gives the probability conditional on a subject having
survived to time T. The death density function is always nonnegative (greater
than or equal to 0), and a peak in the function indicates a time at which the
probability of failure is high.
Other names for the death density function are probability density
function and unconditional failure rate. Related functions are the
hazard function, the conditional instantaneous
probability of the event (failure) given survival up to that time; and the
survival function, which represents the
probability that the event (failure) has not yet occurred. The cumulative
hazard function is the integral over time of the hazard function, and is
estimated as the negative logarithm of the survival function.
- distribution function:
- A distribution function (also known as the probability distribution
function) of a continuous random variable X is
a mathematical relation that gives for each number x, the probability that the
value of X is less than or equal to x. For example, a distribution function of
height gives, for each possible value of height, the probability that the
height is less than or equal to that value. For discrete
random variables, the distribution function is
often given as the probability associated with each possible discrete value of
the random variable; for instance, the distribution function for a fair coin
is that the probability of heads is 0.5 and the probability of tails is 0.5.
- distribution-free tests:
- Distribution-free tests are tests whose validity under the null hypothesis
does not require a specification of the population
distribution(s) from which the data have been
sampled.
- expected cell
frequencies:
- For nominal (categorical) data in which the count of items in each
category has been tabulated, the observed frequency is the actual
count, and the expected frequency is the count predicted by the
theoretical distribution underlying the data. For
example, if the hypothesis is that a certain plant has yellow flowers 3/4 of
the time and white flowers 1/4 of the time, then for 100 plants, the expected
frequencies will be 75 for yellow and 25 for white. The observed frequencies
will be the actual counts for 100 plants (say, 73 and 27).
- factors:
- A factor is a single discrete classification scheme for data, such that
each item classified belongs to exactly one class (level)
for that classification scheme. For example, in a drug experiment involving
rats, sex (with levels male and female) or drug
received could be factors. A
one-way analysis of variance involves a single factor classifying the
subjects (e.g., drug received);
multi-factor analysis of variance involves multiple factors classifying
the subjects (e.g., sex and drug received).
- fixed effects:
- In an experiment using a fixed-effect design, the results of the
experiment apply only to the populations included in the experiment. Those
populations include all (or at least most of) those of interest. This is true
for many experiments, where the effects are due to such variables as gender,
age categories, disease states, or treatments. When the populations included
in the experiment are a random subset of those of interest, then the
experiment follows a random-effects design.
Multiple comparisons tests for an
analysis of variance may be applied when the effects are fixed. They are not
appropriate if the effects are random.
Whether an effect is considered random or fixed may depend on the
circumstances. A factory may conduct an experiment comparing the output of
several machines. If those machines are the only ones of interest (because
they constitute the entire set of machines owned by that company), then
machine will be a fixed effect. If the machines were instead selected randomly
from among those owned by the company, then machine would be a random effect.
- Fisher's exact test:
-
Fisher's exact test for a 2x2 contingency table
is a test of the null hypothesis that the row
classification factor and the column classification
factor are independent.
Fisher's exact test consists of calculating the actual (hypergeometric)
probability of the observed 2x2 contingency table with respect to all other
possible 2x2 contingency tables with the same column and row totals. The
probabilities of all such tables that are each no more likely than the
observed table are calculated. The sum of these probabilities is the P value.
If the sum is less than or equal to the specified
significance level, then the null hypothesis
is rejected.
- goodness of fit:
-
Goodness-of-fit tests test the conformity of the observed data's empirical
distribution function with a posited theoretical
distribution function. The chi-square
goodness-of-fit test does this by comparing observed and expected
frequency counts. The
Kolmogorov-Smirnov test does this by calculating the maximum vertical
distance between the empirical and posited distribution functions.
- hazard function:
- The hazard function is a time to failure
function that gives the instantaneous probability of the event (failure) given
that it has not yet occurred. That is, in a survival experiment where the
event is death, the value of the hazard function at time T is the
probability that a subject will die precisely at time T, given that the
subject has survived to time T. The function may increase with time, meaning
that the longer subjects survive, the more likely it becomes that they will
die shortly (as for cancer patients who do not respond to treatment). It may
decrease with time, meaning that the longer subjects survive, the more likely
it is that they will survive into the near future (as for post-operative
survival for gunshot victims). It may remain constant, as for a population
with a (negative) exponential survival distribution. Or it may have a more
complicated shape, like the well-known "bathtub" curve for human mortality,
where the hazard is high for newborns, drops quickly, stays low through
adulthood, and then rises again in old age.
Other names for the hazard function are instantaneous failure rate,
force of mortality, conditional mortality rate, and
age-specific failure rate. Related functions are the
death density function, the
unconditional instantaneous probability of the event (failure); and the
survival function, which represents the
probability that the event (failure) has not yet occurred. The cumulative
hazard function is the integral over time of the hazard function, and is
estimated as the negative logarithm of the survival function.
- heavy-tailed:
- A heavy-tailed distribution is one in which
the extreme portion of the distribution (the part farthest away from the
median) spreads out further relative to the width of the center (middle 50%)
of the distribution than is the case for the
normal distribution. For a symmetric heavy-tailed distribution like the
Cauchy distribution, the probability of observing a value far from the median
in either direction is greater than it would be for the normal distribution.
Boxplots may help in detecting
heavy-tailedness; normal probability plots
may also help in detecting
heavy-tailedness.
- histogram:
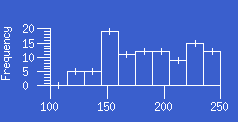
A histogram is a graph of grouped (binned) data in which the number of values
in each bin is represented by the area of a rectangular box.
- homoscedasticity (homogeneity
of variance):
- Normal-theory-based tests for the equality of population means such as the
t test and analysis of variance, assume that the data come from
populations that have the same variance, even if the
test rejects the null hypothesis of equality of
population means. If this assumption of homogeneity of variance is not
met, the statistical test results may not be valid. Heteroscedasticity
refers to lack of homogeneity of variances.
- (in)appropriate use of
chi-square test:
- Pearson's chi-square test for independence for a
contingency table involves using a normal
approximation to the actual distribution of the
frequencies in the contingency table. This approximation becomes less reliable
when the expected frequencies for the
contingency table are very small. A standard (and conservative) rule of thumb
(due to Cochran) is to avoid using the chi-square test for contingency tables
with expected cell frequencies less than 1, or when more than 20% of the
contingency table cells have expected cell frequencies less than 5. In such
cases, an alternate test like Fisher's exact test for a
2x2 contingency table should be considered for a more accurate evaluation of
the data.
- independent:
- Two random variables are independent if
their joint probability density is the product of their individual (marginal)
probability densities. Less technically, if two random variables A and B are
independent, then the probability of any given value of A is unchanged by
knowledge of the value of B. A sample of mutually
independent random variables is an independent sample.
- index plot:
- An index plot of data values is a plot of each value (Y) against its order
in the data set (X). If data are entered into a table in the order in which
they are collected, for example, then a plot of data value against row number
will produce an index plot. An index plot may help detect
correlation between successive data values, a sign
of lack of independence.
- interaction:
- In
multi-factor analysis of variance, factors A and B interact if the effect
of factor A is not independent of the level of
factor B. For example, in an drug experiment involving rats, there would be an
interaction between the factors sex and treatment if the effect
of treatment was not the same for males and females.
- kurtosis:
- Kurtosis is a measure of the heaviness of the tails in a
distribution, relative to the
normal distribution. A distribution with
negative kurtosis (such as the uniform distribution) is
light-tailed relative to the normal distribution,
while a distribution with positive kurtosis (such as the Cauchy distribution)
is heavy-tailed relative to the normal
distribution.
- levels within factors:
- When a factor is used to classify subjects, each
subject is assigned to one class value; e.g., male or female for the factor
sex or the specific treatment given for the factor treatment. These
individual class values within a factor are called levels. Each subject is
assigned to exactly one level for each factor.
Each unique combination of levels for each factor is a cell.
- leverage:
- Leverage is a measure of the amount of influence a given data value has on
a fitted linear regression. For a change in
an observed Y value, the leverage is the proportional change in the fitted Y
value.
- life table method:
- For
survival studies,
life tables are constructed by partitioning time into intervals (usually
equal intervals), and then counting for each time interval: the number of
subjects alive at the start of the interval, the number who die during the
interval, and the number who are lost to follow-up or withdrawn during the
interval. Those lost or withdrawn are censored. Those
alive at the end of a time interval were at risk for the entire
interval. Under the usual actuarial method of
survival function estimation for life tables, the estimate of the
probability of survival within each time interval is calculated by assuming
that any values censored in that interval were at risk for half the interval.
Death can be replaced by any other identifiable event. Unlike the
Kaplan-Meier product-limit method, the life table
survival estimate can still be calculated even if the exact survival or
censoring times are not known for each individual, as long as the number of
individuals who die or are censored within each time interval is known.
- light-tailed:
- A light-tailed distribution is one in which
the extreme portion of the distribution (the part farthest away from the
median) spreads out less far relative to the width of the center (middle 50%)
of the distribution than is the case for the
normal distribution. For a symmetric light-tailed distribution like the
uniform distribution, the probability of observing a value far from the median
in either direction is smaller than it would be for the normal distribution.
Boxplots may help in detecting
light-tailedness; normal probability plots
may also help in detecting
light-tailedness.
- linear functions:
- A linear function of one or more X variables is a linear combination of
the values of the variables:
Y = b0 + b1*X1 + b2*X2 + ... + bk*Xk.
An X variable in the equation could be a curvilinear function of an observed
variable (e.g., one might measure distance, but think of distance squared as
an X variable in the model, or X2 might be the square of X1), as long as the
overall function (Y) remains a sum of terms that are each an X variable
multiplied by a coefficient (i.e., the function Y is linear in the
coefficients). Sometimes, an apparently nonlinear function can be made linear
by a transformation of Y, such as the
function
Y = exp(b0 + b1*X1),
which can be made a linear function by taking the logarithm of Y
(log(Y) = b0 + b1*X1),
and then considering log(Y) to be the overall function.
- linear logistic model:
- A linear logistic model assumes that for each possible set of values for
the independent (X) variables, there is a probability p that an event (success)
occurs. Then the model is that Y is a linear combination of the values of the
X variables:
Y = b0 + b1*X1 + b2*X2 + ... + bk*Xk,
where Y is the logit tranformation of the probability
p.
- linear regression:
- In a linear regression, the fitted (predicted) value of the
response variable Y is a linear combination of the values of one or more
predictor (X) variables:
fitted Y = b0 + b1*X1 + b2*X2 + ... + bk*Xk.
An X variable in the model equation could be a nonlinear function of an
observed variable (e.g., one might observe distance, but use distance squared
as an X variable in the model, or X2 might be the square of X1), as long as
the fitted Y remains a sum of terms that are each an X variable multiplied by
a coefficient. The most basic linear regression model is
simple linear regression, which involves one X variable:
fitted Y = b0 + b1*X.
Multiple linear regression refers to a linear regression with more
than one X variable.
- location:
- The generalized concept of the "average" value of a
distribution. Typical
measures
of location are the mean, the median, the mode, and the geometric mean.
- logit transformation:
- The logit transformation Y of a probabilty p
of an event is the logarithm of the ratio between the probability that the
event occurs and the probability that the event does not occur:
Y = log(p/(1-p)).
- log-rank test:
- In
survival analysis, a log-rank test
compares the equality of k survival functions by creating a sequence of
kx2 contingency tables (k survival functions by
event observed/event not observed at that time) one at each
(uncensored) observed event time, and calculating a
statistic based on the observed and expected values for these contingency
tables. This test is also known as the Mantel-Cox (Mantel-Haenszel)
test. The Tarone-Ware and Gehan-Breslow tests are weighted
variants of the log-rank test; the Peto and Peto log-rank test involves a
different generalization of this log-rank scheme.
- matched samples:
- Matching, also known as pairing (with two samples) and blocking
(with multiple samples) involves matching up individuals in the samples so as
to minimize their dissimilarity except in the factor(s)
under study. For example, in pre-test/post-test studies, each subject is
paired (matched) with himself, so that the difference between the pre-test and
post-test responses can be attributed to the change caused by taking the test,
and not to differences between the individuals taking the test. A study
involving animals might be blocked by matching up animals from the same litter
or from the same cage. The goal is to minimize the variation within the pairs
or blocks while maximizing the variation between them. This will minimize
variation between subjects that is not attributable to the factors under study
by attributing it to the blocking factor. The matched items in a pair or in a
block are related by their membership in that pair or block. Other methods for
controlling for variation between subjects for variables that are not of
direct interest are stratification and the use
of covariates.
- method of maximum likelihood:
- The method of maximum likelihood is a general method of finding estimated
(fitted) values of parameters. Estimates are found such that the joint
likelihood function, the product of the values of the distribution function
for each observed data value, is as large as possible. The estimation process
involves considering the observed data values as constants and the parameter
to be estimated as a variable, and then using differentiation to find the
value of the parameter that maximizes the likelihood function.
The maximum likelihood method works best for large samples, where it tends
to produce estimators with the smallest possible variance. The maximum
likelihood estimators are often biased in small samples.
The maximum likelihood estimates for the slope and intercept in
simple linear regression, are the same as the
least squares estimates when the underlying
distribution for Y is normal. In this case, the maximum likelihood estimators
are thus unbiased. In general, however, the maximum likelihood and least
squares estimates need not be the same.
- measures of association:
- For cross-tabulated data in a contingency table,
a measure of association measures the degree of association between the row
and column classification variables.
Measures of association include the coefficient of contingency,
Cramer's V, Kendall's tau-B, Kendall's tau-C, gamma,
and Spearman's rho,
- method of least squares:
- The method of least squares is a general method of finding estimated (fitted)
values of parameters. Estimates are found such that the sum of the squared
differences between the fitted values and the corresponding observed values is
as small as possible. In the case of simple
linear regression, this means placing the fitted line such that the sum of
the squares vertical distances between the observed points and the fitted line
is minimized.
- median:
- The median of a distribution is the value X such that the probability of
an observation from the distribution being below X is the same as the
probability of the observation being above X. For a continuous distribution,
this is the same as the value X such that the probability of an observation
being less than or equal to X is 0.5.
- median remaining lifetime:
- For
survival studies using life tables, the median
remaining lifetime for an interval of the life table is the estimate of the
additional elapsed time before only half the individuals alive at the
beginning of current interval are still alive. This is also known as the
median residual lifetime.
- mixed models:
- Factors in an analysis of variance (ANOVA) may be either
fixed or random.
Multi-factor ANOVA models in which at least one effect is fixed and at least
one effect is random are called mixed models, especially a two-factor
factorial ANOVA in which one factor is fixed and the other is random. A
randomized block ANOVA is also usually
a mixed model, since the factor of interest is usually a fixed effect.
For two-factor factorial ANOVA, a mixed model is also referred to as a Type
III model. (If both effects are fixed, it's a Type I model, and if both
effects are random, it's a Type II model.)
Sometimes, the term mixed model is also applied to ANOVA models in which at
least one factor is a repeated measures (within)
factor, and at least one factor is a grouping (between)
factor.
- mixture distribution:
- A mixture distribution is a distribution for
which observed values can come from one of multiple distributions. For example,
in taking measurements of blood pressure from a population, the distribution
for males may be a normal distribution, the
distribution for females may also be a normal distribution, but if the two
normal distributions do not have the same mean and variance, then the
composite distribution is not normal.
- multicollinearity:
- In a multiple regression with more than one X
variable, two or more X variables are collinear if they are nearly linear
combinations of each other. Multicollinearity can make the calculations
required for the regression unstable, or even impossible. It can also produce
unexpectedly large estimated standard errors for the coefficients of the X
variables involved. Multicollinearity is also known as collinearity and
ill conditioning.
- multiple comparisons:
- An analysis of variance F test for a specific factor tests the hypothesis
that all the level means are the same for that factor. However, if the null
hypothesis is rejected, the F test does not give information as to which level
means differ from which other level means.
Multiplicity issues make doing individual tests to compare each pair of
means inappropriate unless the nominal (comparisonwise)
significance level is adjusted to account
for the number of pairs (as in a Bonferroni method). An alternative approach
is to devise a test (such as Tukey's test) specifically designed to keep the
overall (experimentwise) significance level at the desired value while
allowing for the comparison of all possible pairs of means. This is a multiple
comparisons test.
- multiple regression:
- Multiple regression refers to a regression model in which the fitted value
of the response variable Y is a function of the values of one or more
predictor (X) variables. The most common form of multiple regression is
multiple linear regression, a linear
regression model with more than one X variable.
- multiplicity of
testing:
- Even when the null hypothesis is true, a
statistical hypothesis test has a small probability (the preselected
alpha-level or significance level) of
falsely rejecting the null hypothesis. With a significance level of 0.05, this
could be considered as the probability of seeing 20 come up on a 20-sided fair
die. If multiple tests are done (the die is rolled multiple times), even if
the null hypothesis in each case is true, the probability of getting at least
one such false rejection (seeing 20 turn up at least once) increases. For the
common problem of comparing pairwise mean
differences following an analysis of variance, the probability of seeing
at least one such false rejection could approach 90% when there are 10 level
means in the factor. To avoid the multiplicity problem, multiple comparison
tests have been devised to allow for simultaneous inference about all the
pairwise comparisons while maintaining the desired
significance level.
- multi-sample problem:
- In the multi-sample problem, multiple independent
random samples are collected, and then the samples are used to test a
hypothesis about the populations from which the
samples came (e.g., whether the means of the populations are all identical).
- nonlinear functions:
- A nonlinear function is one that is not a linear
function, and can not be made into a linear function by
transforming the Y variable.
- nonlinear regression:
- In a
nonlinear regression, the fitted (predicted) value of the response
variable is a nonlinear function of one or more X
variables.
- nonparametric tests:
-
Nonparametric tests are tests that do not make
distributional assumptions, particularly the usual distributional
assumptions of the normal-theory based tests. These include tests that do not
involve population parameters at all (truly
nonparametric tests such as the chi-square goodness
of fit test), and distribution-free tests, whose
validity does not depend on the population distribution(s) from which the data
have been sampled. In particular, nonparametric
tests usually drop the assumption that the data come from
normally distributed populations. However,
distribution-free tests generally do make some assumptions, such as
equality of population variances.
- normal (Gaussian)
distribution:
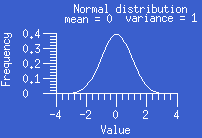
The normal or Gaussian distribution is a continuous symmetric
distribution that follows the familiar bell-shaped
curve. The distribution is uniquely determined by its mean and variance. It
has been noted empirically that many measurement variables have distributions
that are at least approximately normal. Even when a distribution is nonnormal,
the distribution of the mean of many independent observations from the same
distribution becomes arbitrarily close to a normal distribution as the number
of observations grows large. Many frequently used statistical tests make the
assumption that the data come from a normal distribution.
- normal probability plot:
A normal probability plot, also known as a normal Q-Q plot or normal
quantile-quantile plot, is the plot of the ordered data values (as Y)
against the associated quantiles of the normal
distribution (as X). For data from a normal distribution, the points of
the plot should lie close to a straight line.
Examples of these plots illustrate various situations.
- null hypothesis:
- The null hypothesis for a statistical test is the assumption that the test
uses for calculating the probability of observing a result at least as extreme
as the one that occurs in the data at hand. For the
two-sample unpaired t test, the null hypothesis is that the two
population means are equal, and the t test involves
finding the probability of observing a t statistic at least as extreme as the
one calculated from the data, assuming the null hypothesis is true.
- one-sample problem:
- In the one-sample problem, an independent random
sample is collected, and then that sample is used to test a hypothesis
about the population from which the sample came
(e.g., whether the mean of the population is 0, or any other fixed constant
chosen in advance). Paired samples are usually reduced
to a one-sample problem by replacing each pair of responses by the difference
between them (e.g., in a pre-test/post-test experiment, recording the change
from pre-test to post-test).
- order statistics:
- If the data values in a sample are sorted into increasing order, then the
ith order statistic is the ith largest data value. For a sample
of size N, common order statistics are the extremes, the minimum (first
order statistic) and maximum (Nth order statistic). Quantiles or
percentiles such as the median are also calculated from
order statistics.
- outliers:
- Outliers are anomalous values in the data. They may be due to recording
errors, which may be correctable, or they may be due to the
sample not being entirely from the same
population. Apparent outliers may also be due to the
values being from the same, but nonnormal
(in particular, heavy-tailed), population
distribution.
- P value:
- In a statistical hypothesis test, the P value is the probability of
observing a test statistic at least as extreme as the value actually observed,
assuming that the null hypothesis is true. This
probability is then compared to the pre-selected
significance level of the test. If the P value is smaller than the
significance level, the null hypothesis is rejected, and the test result is
termed significant.
The P value depends on both the null hypothesis and the
alternative hypothesis. In particular, a
test with a one-sided alternative hypothesis will generally have a lower P
value (and thus be more likely to be significant) than a test with a two-sided
alternative hypothesis. However, one-sided tests require more stringent
assumptions than two-sided tests. They should only be used when those
assumptions apply.
- paired samples:
- Pairing involves matching up individuals in two samples so as to minimize
their dissimilarity except in the factor under study.
For example, in pre-test/post-test studies, each subject is paired (matched)
with himself, so that the difference between the pre-test and post-test
responses can be attributed to the change caused by taking the test, and not
to differences between the individuals taking the test. Such data are analyzed
by examining the paired differences.
- parallelism assumption:
- For
analysis of covariance (ANCOVA), it is assumed that the
populations can each be correctly modeled by a
straight-line simple linear regression. The
parallelism assumption is that the regressions all have the same slope.
The assumption can be tested by a test of equality for slopes. If the
assumption of equality of slopes does not hold, then a subsequent test of
equality of intercepts (elevations) is meaningless, since it requires that the
slopes be equal.
- pooled
estimate of the variance:
- The pooled estimate of the variance is a weighted average of each
individual sample's variance estimate. When the
estimates are all estimates of the same variance (i.e., when the
population variances are equal), then the pooled
estimate is more accurate than any of the the individual estimates.
- population:
- The population is the universe of all the objects from which a
sample could be drawn for an experiment. If a
representative random sample is chosen, the results of the experiment should
be generalizable to the population from which the sample was drawn, but not
necessarily to a larger population. For example, the results of medical
studies on males may not be generalizable for females.
- power:
- The power of a test is the probability of (correctly) rejecting the
null hypothesis when it is in fact false. The
power depends on the significance level (alpha-level)
of the test, the components of the calculation of the test statistic, and on
the specific alternative hypothesis
under consideration. For the
two-sample unpaired t test, an alternative hypothesis would be that the
difference between the two population means was some
specific non-zero value, such as 1.5; the components of the test statistic
include the sample sizes, sample means, and sample variances. The greater the
power of a two-sample unpaired t test, the better able it is to correctly
reject (i.e., declare significant) small but real differences between the two
population means. A power curve plots the power against the actual
difference between the population means.
- product-limit method:
- For survival studies, the product-limit (Kaplan-Meier)
estimate of survival is calculated by dividing time into intervals such that
each interval ends at the time of an observation, whether
censored or uncensored. The probability of survival is calculated at the
end of each interval, with censored observations assumed to have occurred just
after uncensored ones. The product-limit survival function is a step function
that changes value at each time point associated with an uncensored value.
- qualitative:
- Qualitative variables are variables for which an attribute or
classification is measured. Examples of qualitative variables are gender or
disease state.
- quantitative:
- Quantitative variables are variables for which a numeric value
representing an amount is measured.
- random effects:
- When the populations included in an experiment are a random subset of
those of interest, then the experiment follows a random-effects design. In a
experiment using a random-effects design, the results of the experiment apply
not only to the populations included in the experiment, but to the wider set
of populations from which the subset was taken. For example, subjects in a
repeated measures (within factors) design are
considered a random effect because we are interested not in the particular
subjects chosen for the experiment, but the entire population of potential
subjects. Similarly, blocks are often a random effect in analysis of variance.
Multiple comparisons tests for an
analysis of variance are not applied when the effects are random.
Whether an effect is to considered random or fixed
may depend on the circumstances. A factory may conduct an experiment comparing
the output of several machines. If those machines are the only ones of
interest (because they constitute the entire set of machines owned by that
company), then machine will be a fixed effect. If the machines were instead
selected randomly from among those owned by the company, then machine would be
a random effect.
- random sample:
- A random sample of size N is a collection of N objects that are
independent and identically
distributed. In a random sample, each member of the
population has an equal chance of becoming part of the sample.
- random variable:
- A random variable is a rule that assigns a value to each possible outcome
of an experiment. For example, if an experiment involves measuring the height
of people, then each person who could be a subject of the experiment has
associated value, his or her height. A random variable may be discrete
(the possible outcomes are finite, as in tossing a coin) or continuous
(the values can take any possible value along a range, as in height
measurements).
- randomized block
design:
- A randomized block analysis of variance design such as
one-way blocked ANOVA is created by first grouping the experimental
subjects into blocks such that the subjects in
each block are as similar as possible (e.g., littermates), and there are as
many subjects in each block as there are levels of the factor of interest, and
then randomly assigning a different level of the factor to each member of the
block, such that each level occurs once and only once per block. The blocks
are assumed not to interact with the factor.
- rank tests:
- Rank tests are nonparametric tests that
are calculated by replacing the data by their rank values. Rank tests may also
be applied when the only data available are relative rankings. Examples of
rank
tests include the
Wilcoxon signed rank test, the
Mann-Whitney rank sum test, the
Kruskal-Wallis test, and
Friedman's test.
- repeated measures ANOVA:
- In a repeated measures ANOVA, there will be at least one factor that is
measured at each level for every subject in the experiement. This is a
within (repeated measures) factor. For example,
in an experiment in which each subject performs the same task twice is a
repeated measures design, with trial (or trial number) as the within factor.
If every subject performed the same task twice under each of two conditions,
for a total of 4 observations for each subject, then both trial and condition
would be within factors.
In a repeated measures design, there may also be one or more factors that
are measured at only one level for each subject, such as gender. This type of
factor is a between or grouping factor.
- residuals:
- A residual is the difference between the observed value of a response
measurement and the value that is fitted under the hypothesized model. For
example, in a
two-sample unpaired t test, the fitted value for a measurement is the mean
of the sample from which it came, so the residual would be the observed value
minus the sample mean.
- resistant:
- A statistic is resistant if its value does not change substantially when
an arbitrary change, no matter how large, is made in any small part of the
data. For example, the median is a resistant measure of location, while the
mean is not; the mean can be drastically affected by making a single data
value arbitrarily large, whereas the median can not.
- robust:
- Robust statistical tests are tests that operate well across a wide variety
of distributions. A test can be robust for
validity, meaning that it provides P values close to the true ones in the
presence of (slight) departures from its assumptions. It may also be robust
for efficiency, meaning that it maintains its statistical power (the
probability that a true violation of the null
hypothesis will be detected by the test) in the presence of those
departures.
- scale:
- The generalized concept of the variability or dispersion of a
distribution. Typical
measures
of scale are variance, standard deviation, range, and interquartile range.
Scale and spread both refer to the same
general concept of variability.
- shape:
- The general form of a distribution, often
characterized by its skewness and
kurtosis (heavy or
light tails relative to a normal
distribution).
- significance level:
- The significance level (also known as the alpha-level) of a
statistical test is the pre-selected probability of (incorrectly) rejecting
the null hypothesis when it is in fact true.
Usually a small value such as 0.05 is chosen. If the P
value calculated for a statistical is smaller than the significance level,
the null hypothesis is rejected.
- skewness:
- Skewness is a lack of symmetry in a distribution.
Data from a positively skewed (skewed to the right) distribution have values
that are bunched together below the mean, but have a long tail above the mean.
(Distributions that are forced to be positive, such as annual income, tend to
be skewed to the right.) Data from a negatively skewed (skewed to the left)
distribution have values that are bunched together above the mean, but have a
long tail below the mean. Boxplots may be useful in
detecting skewness to the
right or to the
left; normal probabilty plots may also be
useful in detecting skewness to the
right or to the
left.
- spread:
- The generalized concept of the variability of a
distribution. Typical
measures
of spread are variance, standard deviation, range, and interquartile
range.
Spread and scale both refer to the same
general concept of variability.
- stratification:
- Stratification involves dividing a sample into homogeneous subsamples
based on one or more characteristics of the population. For example, samples
may be stratified by 10-year age groups, so that, for example, all subjects
aged 20 to 29 are in the same age stratum in each group. Like
blocking or the use of
covariates, stratification is often used to control for variation that is
not attributable to the variables under study. Stratification can be done on
data that has already been collected, whereas blocking is usually done by
matching subjects before the data are collected. Potential disadvantages to
stratification are that the number of subjects in a given stratum may not be
uniform across the groups being studied, and that there may be only a small
number of subjects in a particular stratum for a particular group.
- structural zeros:
- The process that creates the observations that appear in a
contingency table may produce
cells in the contingency table in which observations can never occur. The
zero values that must occur in these cells are structural zeroes. For
example, a contingency table of cancer incidence by sex and type of cancer
must have the value 0 in the cell for males and ovarian cancer, but the
expected number of males with ovarian cancer will not be 0 as long as there is
are at least 1 male and 1 ovarian cancer patient among the observations. A
contingency table containing one or more structural zeroes is an incomplete
table. Pearson's chi-square test for independence
and Fisher's exact test are not designed for contingency
tables with structural zeroes.
- survival function:
- The survival function is a time to failure
function that gives the probability that an individual survives (does not
experience an event) past a given time. That is, in a survival experiment
where the event is death, the value of the survival function at time T
is the probability that a subject will die at some time greater than T. The
survival function always has a value between 0 and 1 inclusive, and is
nonincreasing. The function is used to find percentiles for survival time, and
to compare the survival experience of two or more groups.
The mortality function is simply 1 minus the survival function.
Other names for the survival function are survivorship function and
cumulative survival rate. Related functions are the
hazard function, the conditional instantaneous
probability of the event (failure) given survival up to that time; and the
death density function, which represents
the unconditional probability that the event occurs exactly at time t. Steeper
survival curves (faster drop off toward 0) suggest larger values for the
hazard or death density functions, and shorter survival times. The
cumulative hazard function is the integral over time of the hazard
function, and is estimated as the negative logarithm of the survival function.
- test of independence:
- A test of independence for a contingency table
tests the null hypothesis that the row
classification factor and the column classification
factor are independent. Two
such tests are Pearson's chi-square test for independence
and Fisher's exact test.
- time to failure distributions:
- In
survival analysis, data is collected on the time until an event is
observed (or censoring occurs). Often this event is
associated with a failure (such as death or cessation of function). The
probability distribution of such times can be
represented by different functions. Three of these are: the
survival function, which represents the
probability that the event (failure) has not yet occurred; the
death density function, which is the
instantaneous probability of the event (failure); and the
hazard function, which is the instantaneous
probability of the event (failure) given that it has not yet occurred. The
cumulative hazard function is the integral over time of the hazard
function, and is estimated as the negative logarithm of the survival function.
- transformation:
- A transformation of data values is done by applying the same function to
each data value, such as by taking logarithms of the data.
- truncated distribution:
- A distribution is truncated if observed values
must fall within a restricted range, instead of the expected range over all
possible real values. For example, a observation from a
normal distribution can take any real value
between -infinity and +infinity. An observation from a truncated normal
distribution might only take on values greater than 0, or less than 2.
- two-sample problem:
- In the two-sample problem, two independent random
samples are collected, and then the samples are used to test a hypothesis
about the populations from which the samples came
(e.g., whether the means of the two populations are identical).
- two-way layout:
- The two-way layout refers to a two-way classification in which there are
two factors affecting the observed response
measurements. Each possible combination of levels from both factors is
observed, usually once each. The interaction
between the two factors is generally assumed to be 0. The
randomized block design is one example
of a two-way layout.
- violation of assumptions:
- Statistical hypothesis tests generally make assumptions about the
population(s) from which the data were
sampled. For example, many normal-theory-based
tests such as the
t test
and
ANOVA assume that the data are sampled from one or more
normal distributions, as well as that the
variances of the different populations are the same (homoscedasticity:).
If test assumptions are violated, the test results may not be valid.
-
Welch-Satterthwaite t test:
- The
Welch-Satterthwaite t test is an alternative to the
pooled-variance t test, and is
used when the assumption that the two populations
have equal variances seems unreasonable. It provides a t statistic that
asymptotically (that is, as the sample sizes become large) approaches a t
distribution, allowing for an approximate t test
to be calculated when the population variances are not equal.
- within effects:
- In a repeated measures ANOVA, there will
be at least one factor that is measured at each level for every subject. This
is a within (repeated measures) factor. For
example, in an experiment in which each subject performs the same task twice,
trial number is a within factor. There may also be one or more factors that
are measured at only one level for each subject, such as gender. This type of
factor is a between or grouping factor.
|